ASD - not a ‘broken cog’ in the machine: The computational variation behind Autism Spectrum Disorder
- comdepri
- Dec 4, 2024
- 5 min read
Updated: Dec 8, 2024
A simple principle – dynamic range – accounts for known differences in autism, suggesting autism may be rooted in a computational trade-off
Individuals with Autism Spectrum Disorder (ASD) show neural and behavioral differences compared to neurotypicals (NT), yet the computational principles driving these differences are not clear. Our study suggests that changes to a basic property of any computational system (i.e. the brain), namely dynamic range, can explain a wide range of phenomena seen in individuals diagnosed with autism spectrum disorder. Our view defies previous descriptions of ASD as a "broken cog in the machine" and provides a deeper, richer account of the computational basis of autism spectrum disorder.
What is Dynamic range? Dynamic range refers to the range of input signal values to which the sensing system responds. If the system’s response changes sharply over a narrow range of signal values, the dynamic range is small. Conversely, if the response changes gradually over a broad range of signal values, the dynamic range is large. In this study, we model the response of a neuron to its input using a sigmoidal function:

In this formulation, S is the signal (between 0 and 1), K is the half-activation point, meaning the signal value for which the response reaches half of the maximum activity level, and n - the slope of the sigmoid. The dynamic range is determined by the variable n, termed the “Hill coefficient”. The smaller it is – the more gradual the function, meaning a larger dynamic range. Dynamic range is an interesting principle to study, as it is fundamental to any information processing system and has (as will be shown below) profound effects on the possible computations a system can perform. In the next few hundred words, we’ll walk through how increased dynamic range (IDR) resembles ASD-like behavior and computations.
Increased Dynamic Range allows for better discrimination between close-by input signal values away from the middle of the signal range
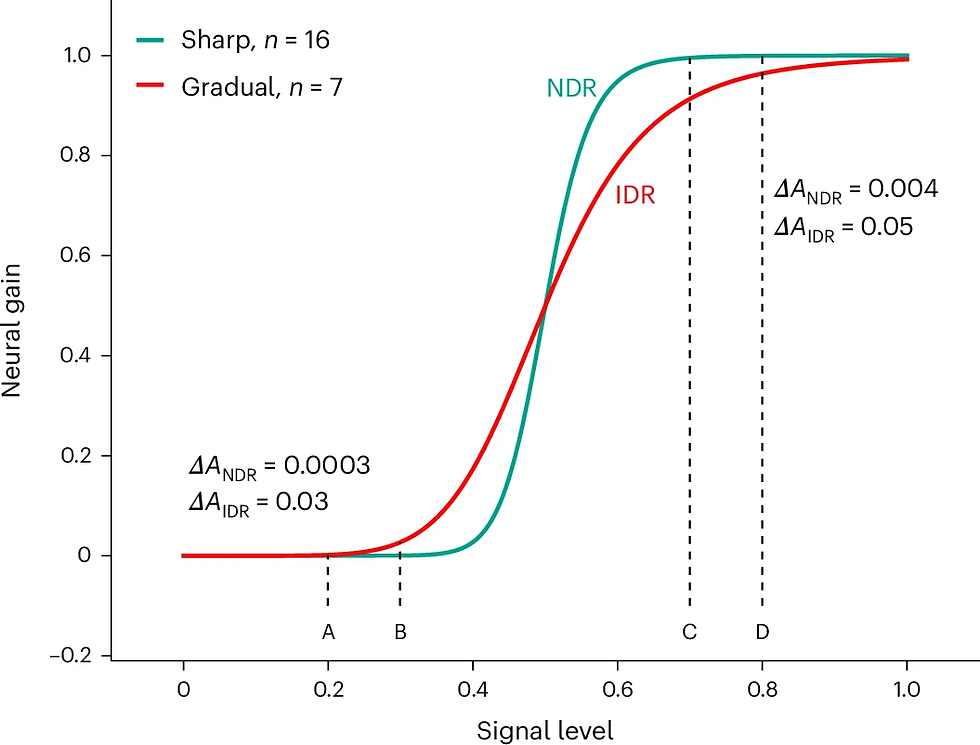
Individuals diagnosed with autism spectrum disorder show increased discrimination between two close-by signals in a multitude of perceptual tasks, for example search ability for small elements.
This heightened sensitivity comes naturally with increased dynamic range. Comparing between a sharp and a gradual population responses, shows this very clearly. A gradual population response (red line) for close-by signals has discernible responses, allowing distinct decoding of the two input signals (B vs A, D vs C). This heightened sensitivity is not available for the sharp population response (turquoise line) because close-by signals far from the half-activation point () elicit almost identical responses.
Increased dynamic range increases the range of elevated neural variability
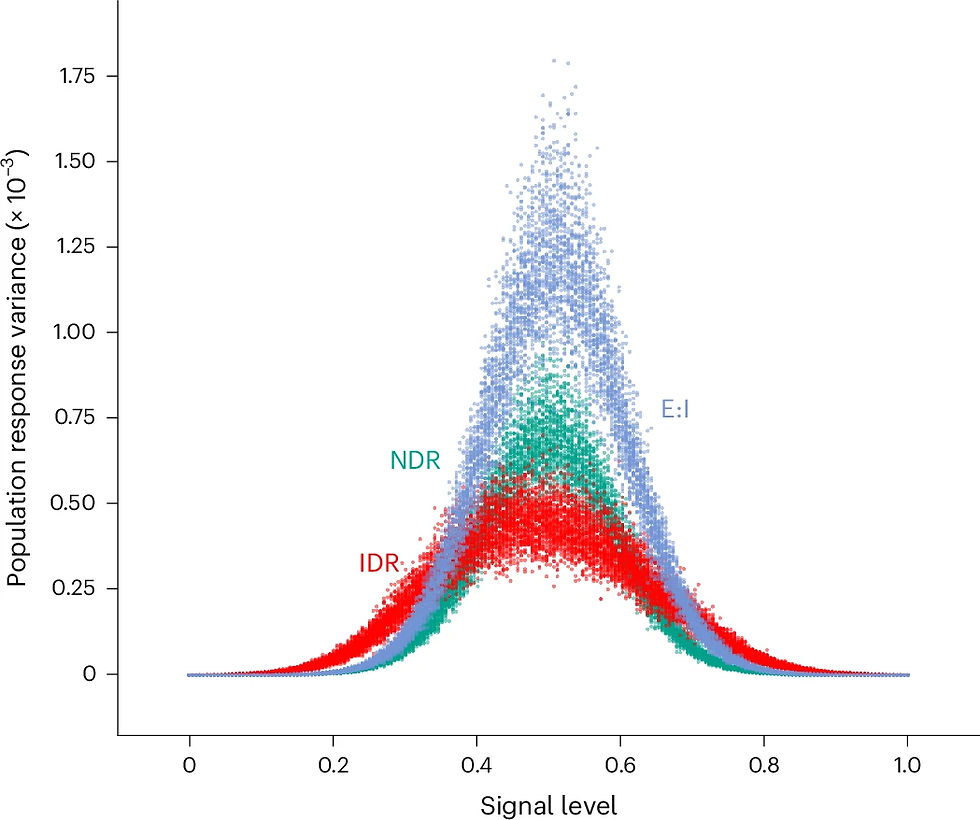
One well studied observation is that individuals with autism spectrum disorder show increased variance in brain measurements, such as EEG or fMRI. One of the prominent current models for autism spectrum disorder is that of increased excitation to inhibition ratio (E:I model). This model posits that autism spectrum disorder is a result of detrimentally more excitation relative to inhibition in neural circuits. Here, we comparing the gradual response (IDR) to the sharp response (NDR and E:I) models and found that the interval of notable variance is wider for the gradual response compared with the sharp response model and the E:I model. This provides an avenue for contrasting the increased dynamic range (IDR) and E:I models – while IDR predicts wider variance curves for ASD, the E:I model predicts similar widths for both ASD and NT.
Increased dynamic range entails slower updating rates to abrupt changes
Individuals diagnosed with ASD show slower updating rates to changes in the environment in auditory and motor tasks. For example, in a finger-tapping synchronization task, Vishne et al. (2021) asked participants with and without ASD to synchronize their finger-tapping to a metronome beat. Unbeknownst to the subjects, the frequency of the metronome changed every few beats – speeding up or slowing down. They found that the ASD group followed the metronome beat change more slowly, indicating slower updating of the estimation of the incoming signal. Using a variation of Kalman-Filter, an optimal method to estimate a signal from noisy measurements, our simulations showed how having increased dynamic range slows the update of signal estimates (Panel A). Furthermore, we fit the increased dynamic range (IDR) model to the tapping-synchronization task data, revealing lower Hill-coefficients (n values, the slopes for the encoding function) for the ASD group compared to the NT group (Panel B), with good reproductions of the experimental results (ASD group panel C, NT group panel D). An increased dynamic range means increased variance in the neuronal response over a larger portion of the input signal range which implies lower confidence in the incoming signal, eliciting a smaller update of the prior belief. This gradual response can carry benefits: if the abrupt changes come only from noise, then an IDR with its gradual response will filter them better.
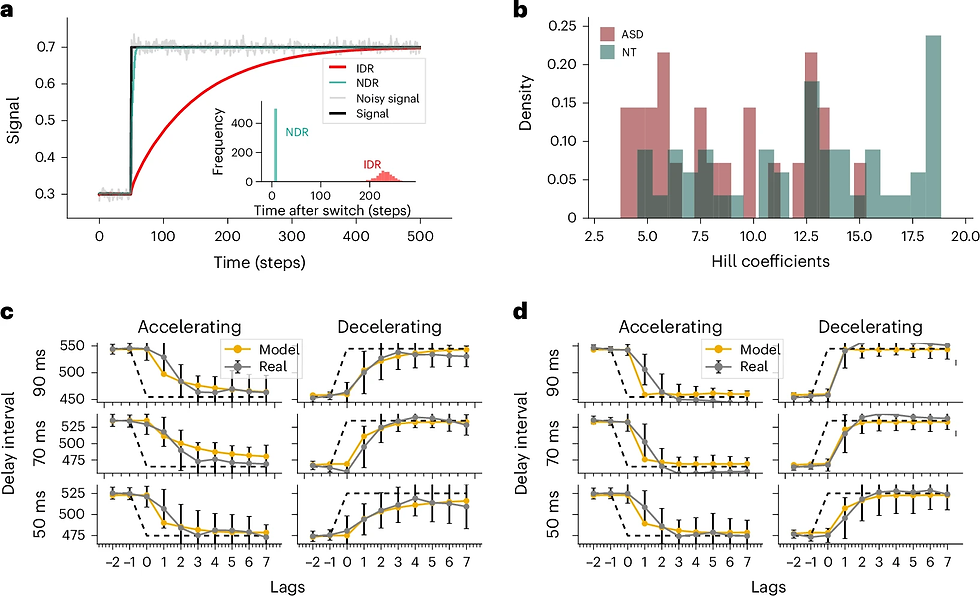
Increased dynamic range changes the encoding scheme reduces total encoding capacity
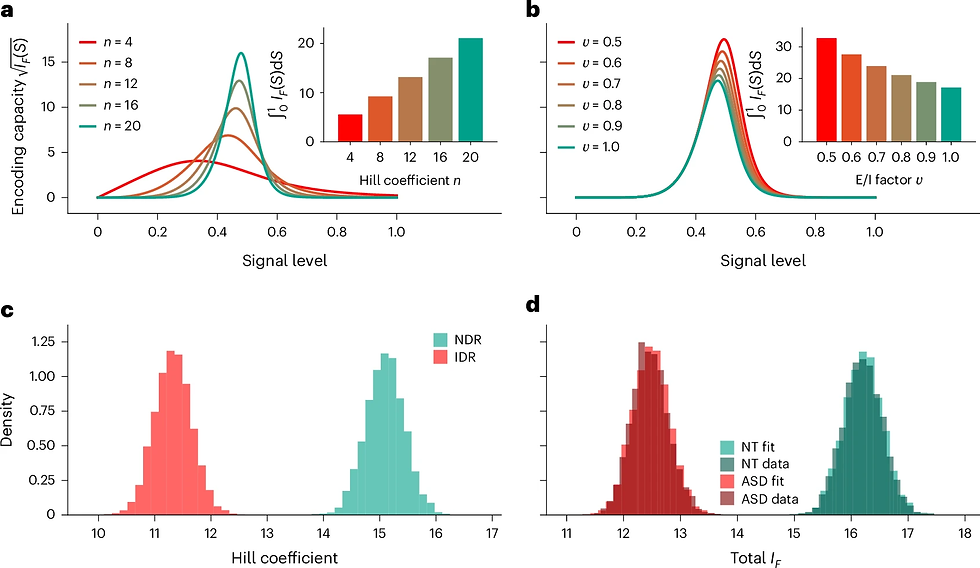
Using concepts from information theory, specifically Fisher Information, we can quantify the amount of information encoded on the signal with different encoding schemes. Varying the Hill coefficient (slope of the encoding) reveals how IDR, contrary to E:I, changes the encoding scheme – spreading encoding resources across the signal range while reducing the overall amount of information encoded (Panel A, IDR and Panel B, E:I). This is in line with results known from the literature (Noel et al. 2021) – fitting these results again reveals lower Hill-coefficients for the ASD group, consistent with the increased dynamic range (IDR) model.
A possible biological mechanism driving increased dynamic range

How might an IDR arise in biology? We propose a biological mechanism based on the heterogeneity of the half-activation point in individual neurons within the same population. If there is more heterogeneity, the average population response would have increased dynamic range. Given the vast literature that maps autism spectrum disorder to mutations in genes related to neuronal receptors and synapses, this proposed biological mechanism is highly relevant—many sources of heterogeneity in these neuronal features can lead to increased dynamic range. This could explain the copious amount of different genes associated with ASD.
In summary, we establish a new computational model for autism spectrum disorder (ASD), positing that ASD is an extreme of a natural computational trade-off between accurate encoding and fast adaptation, based on variations along the spectrum of the dynamic range of neuronal population response. This trade-off highlights the need to understand the benefits and costs of the different computation schemes. Broadly, the increased dynamic range model of ASD offers a new computational principle, opening avenues for investigating the biological, neural, behavioral and computational aspects of ASD and defying the ‘broken cog’ view of ASD.
Komentarze